The Leading Qualified Medical Device Supplier Directory
Discover + Source + Connect
Discover Qualified Suppliers You Can Trust
With the Qmed+ directory, you can trust you'll be connected with qualified suppliers, service providers, and consultants to the medtech industry that meet global standards for current good manufacturing practice (cGMP) regulations and quality management systems (QMS). In order to be considered for inclusion in the Qmed+ supplier directory, companies must show qualification through these criteria or proven experience working with medical device manufacturers.




Featured Products
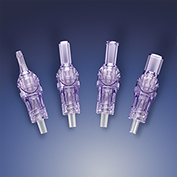
Qosina Adds Closed Male Luer Lock Valves
QosinaQosina has added four closed male luer lock valves to its existing line of luer-activat...
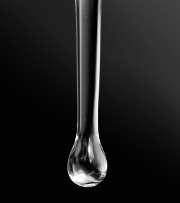
Hydrophilic Coating Services
Teleflex Medical OEMTeleflex Medical OEM offers a proprietary, hydrophilic surface coating for polymer-based devices that are...
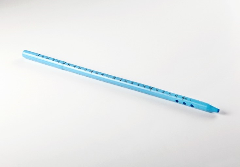
Catheter Lamination
Rose MedicalVariety of complex catheter reflow capabilities including braid and coil reinforcement, variable durometer, complex ID ...
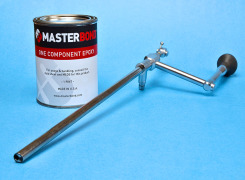
EP3HTMed: Chemically Resistant Polymer Meets USP Class VI Specifications
Master Bond Inc.Master Bond EP3HTMed fully meets USP Cl...
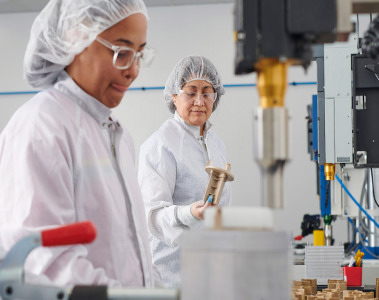
Post-Molding Assembly
Beacon MedTech SolutionsAs your full-service partner, we have both cleanroom and non-cleanroom space for executing your complex assemblies....
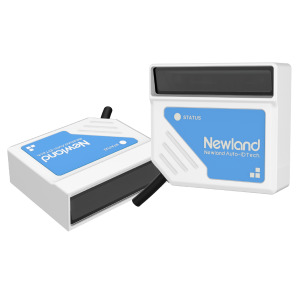
NLS-FM515 Series
Newland AIDC• Support 1D barcodes• Ultra-High Scan Speed and Motion Tolerance: 780 scans per second • Depth of field: FM515-V...
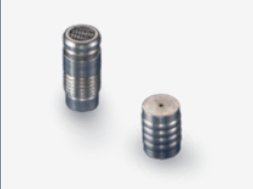
Flow Metering
The Lee CompanyAlso known as restrictor check valves and flow control valves, flow metering valves from The Lee Company are designed to add bot...
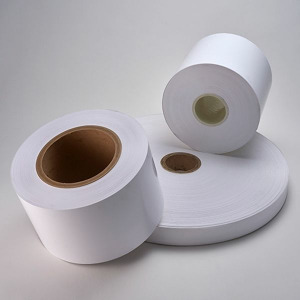
MELINEX® 329 Polyester Film
Tekra, LLCMELINEX® 329 is an opaque white, non-pretreated, polyester film with superb handling properties and a uniqu...
Featured Companies
The Qmed+ directory simplifies and accelerates the new supplier research and discovery process. And it's easy to find exactly what you need: Search suppliers to the medical device industry by keyword or by product or service category.
Within each supplier's directory listing, you'll find a comprehensive company overview, contact information, qualifications, products and capabilities, supplemental resources, and ways to connect.
Discover and contact thousands of pre-qualified suppliers to the medical device and in vitro diagnostics industry. Start your sourcing journey now!
Medical device and diagnostics manufacturing is a fast-moving and highly regulated industry. Today, new and exciting technologies are enabling the creation of innovative medical products that are improving patient care by leaps and bounds. At the same time, the global web of regulatory requirements governing how those products are designed, developed, and produced is growing ever more complex. As a result, it’s becoming less and less likely that individual medical technology manufacturers have all the experience and capabilities they need—from design and development to manufacturing and regulatory affairs—in house.
Medical device manufacturers today can’t do it all themselves, so they must turn to outsourcers—design firms, testing labs, contract manufacturers, component and equipment suppliers, and consulting firms—to help bring their innovations to market. But finding trusted partners in the scattered landscape of medtech suppliers and service providers is no small task.
That’s why we created Qmed, the world’s only directory of pre-qualified suppliers and service providers to the medical device and diagnostics industry. For nearly a decade, Qmed’s powerful search tool and online directory have enabled medtech manufacturers to connect with relevant partners that can help fill the gaps in their current capabilities and help speed their life-saving and -improving medical devices to market.
When medical device manufacturers turn to the Qmed directory for help in finding qualified outsource partners, they can trust they’ll be connected with suppliers, service providers, and consultants that meet global standards for good manufacturing practice and quality control, and which have relevant experience in the complex and evolving medtech industry.
In order to be considered for inclusion in the Qmed directory, companies must show qualification through some of the following criteria:
- ISO 9001 certification
- ISO 13485 certification
- CGMP compliance
- FDA registration
- Demonstrated experience with clients in the medical device or in vitro diagnostics space